Algorithms on social media monitor and collect user data and recommend relevant content. These algorithms determine the relevancy of content i.e., posts, people, groups by optimizing for engagement according to this journal article on Social Drivers and Algorithmic Mechanisms. In this blog post I will explain how algorithms optimize for engagement, the societal implications of algorithmic optimization, and make suggestions about how algorithms can be better engineered to respond to these potential implications.
One implication often spoken about is that how social media algorithms are highly proprietary, and their mechanisms lack transparency according to this article on What is Social Media Algorithm? Engagement can be measured by number of clicks, likes, comments and time overall spent on social media platforms as defined in the article from SAGE Publications Inc about Social Drivers and Algorithmic Mechanisms. Because these algorithms are designed to be highly proprietary the implication is that echo chambers and filter bubbles contribute to social and political polarization and segmentation. Below is a graph showing a rise in the number of articles containing these key terms.
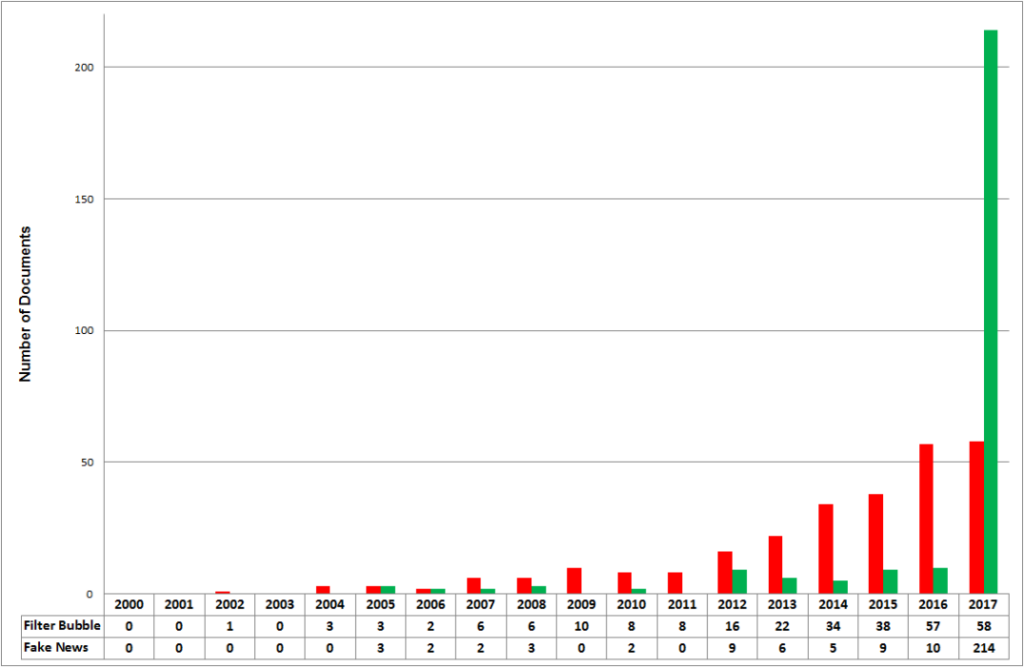
This article on Digital Media Literacy defines an echo chamber as “an environment where a person only encounters information or opinions that reflect and reinforce their own.” According to the article Social Drivers and Algorithmic Mechanisms, algorithms are constantly adapting to user engagement and are updated on social media platforms. Facebook’s algorithm initially optimized content for engagement based on metrics like clicks, likes and comments. It wasn’t long before malicious actors, fake accounts, and spammers figured out how to use these algorithmic metrics to their advantage. So Facebook updated the algorithm to “prioritize social interactions” by giving more weight to content posted by friends and family. In 2015 they added emotional rection buttons and posts that gained reactions were also given more weight.
Engaging content is not always quality content and in fact the same article on Algorithmic Mechanisms states that the more engaging a piece of content was the more it lacked in quality. When Facebook initially added emotional reaction buttons the angry reaction was weighted five times more than the like reaction, favoring lower quality content that elicited strong emotional responses, and was more likely to be partisan or contain falsehoods. In response to concerns, Facebook reduced the weight of the angry response to zero in 2020.
While Facebook and other social media platforms respond to concerns about low quality content by updating algorithmic metrics, this article from Research Gate about Echo Chambers and Filter Bubbles of Fake News in Social Media begs the question, “Are echo chambers and filter bubbles of deceptions and fake news man-made or produced by algorithms?” And the answer is both. This article from Reuters about The Truth Behind Filter Bubbles defines them as “a state of intellectual or ideological isolation that may result from algorithms feeding us information we agree with, based on our past behavior and search history.”
In essence, filter bubbles are more or less the product of engineered algorithmic feedback loops and because these algorithms are complex systems, the creation and perpetuation of filter bubbles is often out of control of engineers to some degree. This is also due to there being vast scales of online social networks in comparison to offline social networks and the proprietary design of algorithms and the content being shown to each individual user. This gives more of an opportunity for exposure to misinformation, low quality content, and falsehoods. An article titled It’s time to stop trusting Facebook to engineer our social world warns that falsehoods tend to spread more quickly across vast social networks because they generate more engagement.
The same article uses the term “business internet” to describe how the business models of social media platforms optimize for engagement, which means they automatically optimize for the spread of falsehoods and other low-quality content.
To curb the spread of falsehoods and misinformation which could be a contributing factor in political and social polarization, Meta, which owns Facebook and Instagram monitors for this type of content by using third-party fact checkers. Posts that contain misleading information are marked by fact checkers and given a lower weight. On Facebook’s website it states that posts are only removed if they explicitly violate community standards. One possible implication of this is that creating and spreading false content can be done in less time than it takes to fact-check. Additionally, Meta prioritizes giving flagged content less weight instead of removing it altogether. It has been speculated that this is because engagement turns a profit, and that removing instead of giving less weight to this kind of content goes against their own business model.
The article Social Drivers and Algorithmic Mechanisms suggest that algorithms can address these implications and be improved by implementing different optimization metrics, or by taking a different approach or additional measures to intervene when false content and disinformation is detected by fact checkers without needing to remove the content or demerit their business models.
One suggestion given is to implement a metric that optimizes for content that is not partisan or as highly proprietary and give more weight to trustworthy moderate news sources and affiliated groups. Secondly, by adding additional time between when a post can be viewed or shared if it is flagged by fact-checkers in addition to giving it a lower weight could also help curb the spread of low-quality information which can be shared and reach many individual users nearly instantaneously because of weighted metrics and the likelihood it will be engaged with.